Artificial intelligence has been a trending topic for several years, constantly making headlines month after month, even week after week. This field is evolving at such a rapid pace that advances follow one another in quick succession. Recently, two approaches have been generating increasing interest: generative AI and agentic AI.
While generative AI has captured public attention with its ability to create impressive content, agentic AI represents a more discreet but potentially more transformative evolution. This article explores the fundamental differences between these two paradigms, while clarifying the often misunderstood relationship between agentic AI and AI agents.
Whether you're a developer, decision-maker, or simply curious about the latest advances in artificial intelligence, this analysis will help you understand how these technologies are redefining our relationship with automated systems and opening new possibilities for innovation.
What is generative AI?
Generative AI represents a category of artificial intelligence systems designed to create original content from the data on which they were trained. These systems, often called large language models (LLMs), primarily use deep neural network architectures, such as transformers, to analyze and learn patterns and structures inherent in vast datasets.
Fundamental principles of generative AI
At the heart of generative AI is the ability to produce new content that didn't exist before but resembles the training data. These models work by identifying recurring patterns and trends in the data (such as sentence structures or frequent word combinations) and creating new original content that follows these same structures. Large language models (LLMs), like GPT or Claude, represent the most advanced example of this approach, capable of predicting and generating text by analyzing the probabilities of word sequences.
Generative AI is fundamentally characterized by its creation orientation, specifically designed to produce content such as text, images, code, music, or videos. Its reactive functioning also distinguishes it, as it primarily responds to user prompts and queries without taking its own initiative. It is furthermore constrained by contextual limitations, only being able to reason about information present in its immediate context. Finally, it is defined by an absence of autonomy, unable to take initiatives or pursue objectives without explicit human instruction.
Common applications of generative AI
Generative AI has found numerous applications across various domains. In the text creation sector, it excels at writing articles, summaries, scripts, or marketing content. For image generation, these technologies allow creating illustrations, designs, or visual concepts from simple textual descriptions. Code development also benefits from these advances, with programming assistance, code generation, and increasingly sophisticated debugging capabilities.
In the artistic domain, musical composition is enriched with new possibilities through the creation of automatically generated melodies, arrangements, or complete pieces. Finally, 3D design and creation benefit from these technologies for the design of models, environments, and characters with remarkable efficiency.
The most well-known examples of generative AI include language models like GPT-4 and Claude, as well as image generators like DALL-E, Midjourney, and Stable Diffusion.
What is agentic AI?
Agentic AI represents a fundamentally different approach to artificial intelligence. Rather than focusing on content creation, agentic AI emphasizes autonomous decision-making and action based on specific objectives.
Definition and principles of agentic AI
Agentic AI refers to artificial intelligence systems designed to operate with a high degree of autonomy, capable of perceiving their environment, making decisions, and acting to achieve predefined objectives, all with minimal human intervention.
The term "agentic" derives from the concept of agency, the ability of a system to act independently in its environment. This approach is radically different from generative AI through its decisional autonomy, allowing it to make decisions without constant human intervention. Its goal orientation is also distinctive, as it actively pursues goals rather than simply responding to instructions.
Adaptability constitutes another fundamental characteristic, allowing strategies to be adjusted according to environmental changes. Through continuous learning, these systems constantly improve their performance through feedback. Finally, their proactivity particularly distinguishes them, as they can initiate actions rather than simply reacting to commands or instructions received.
How agentic AI works
Agentic AI generally functions according to a four-stage cycle, often called the "perceive-reason-act-learn" cycle:
The perception phase constitutes the first pillar of this cycle, where the system collects data from the environment via various sensors or interfaces.
For example, in a financial trading assistant, the AI agent continuously perceives market fluctuations, the latest economic news, analyst reports, and historical data of monitored securities.
Next comes the crucial stage of reasoning, during which the AI analyzes the collected information to understand the situation and plan an appropriate response.
In our trading example, the agent analyzes how a recent central bank announcement might impact certain sectors, evaluates correlations between different indicators, and determines opportunities that match the defined investment criteria.
The action phase represents the moment when the system concretely executes the decisions made during the previous stage.
The financial agent then executes precisely calibrated buy or sell orders according to its strategy, choosing the optimal moment and adjusting volumes to minimize market impact.
Finally, the cycle is completed by learning, where the AI evaluates the results obtained and adjusts its future strategies accordingly.
The trading agent analyzes the performance of its transactions, identifies which strategies succeeded or failed under which market conditions, and progressively refines its models to improve future decisions.
This process often relies on large language models (LLMs) as orchestrators, coordinating various specialized functions and tools to achieve defined objectives.
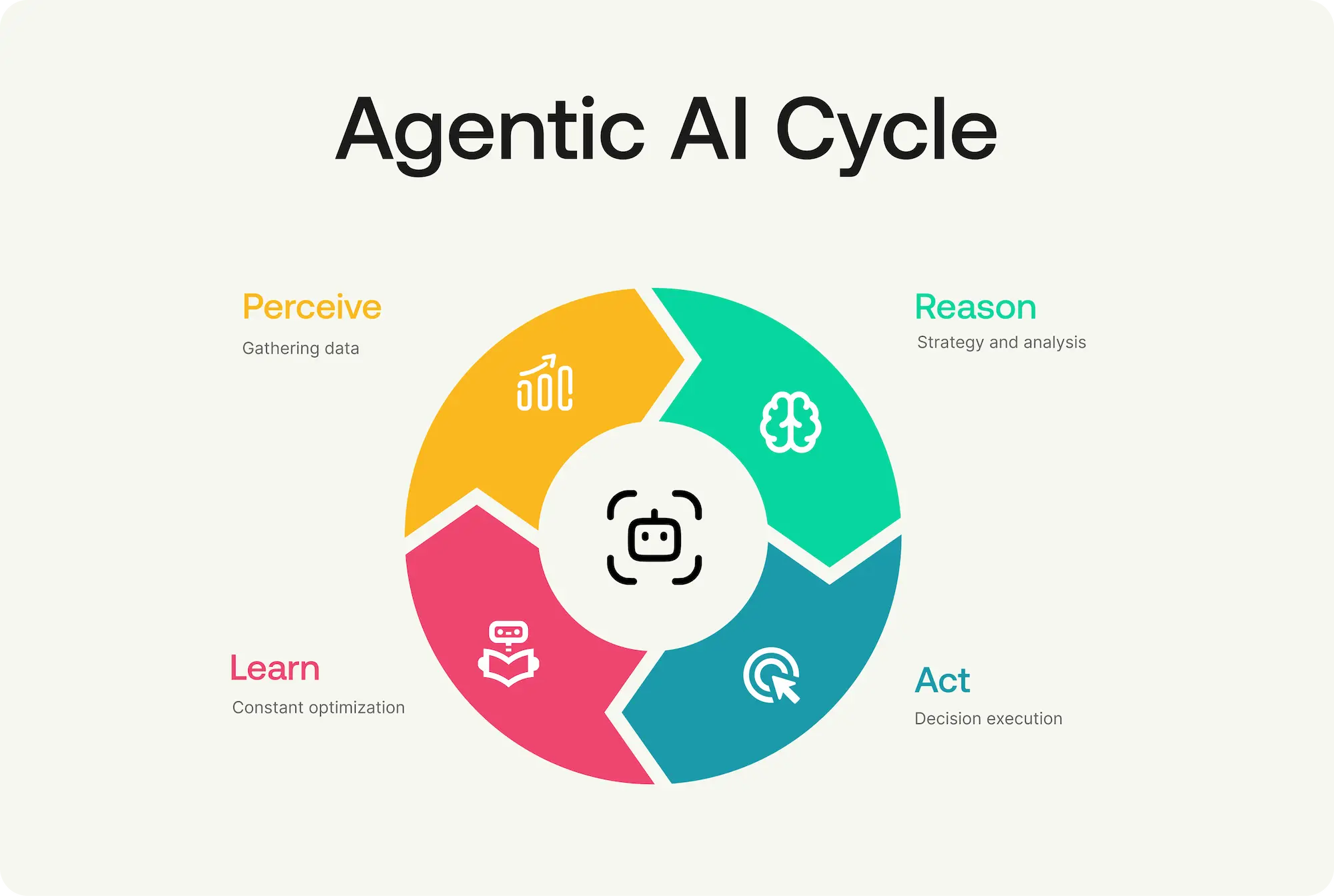
Agentic AI vs. generative AI: key differences
Although generative AI and agentic AI both represent significant advances in the field of artificial intelligence, they differ fundamentally on several crucial aspects.
Comparison table
Fundamental architectural differences
The architecture of agentic AI systems is generally more complex than that of generative systems. It often involves a combination of essential components for optimal functioning.
At the heart of this architecture is a "brain" model that coordinates decisions, typically an LLM serving as a central orchestrator. Sophisticated perception modules complement this brain by collecting varied data from the environment. To execute specific actions, the system integrates specialized tools dedicated to precise tasks.
Information persistence is ensured by memory systems that maintain context across interactions. Finally, reinforcement learning mechanisms allow continuous improvement of system performance over time and through experiences.
In contrast, the architecture of generative AI is primarily centered on a large model trained to maximize the quality of generated content, with little or no capability for interaction with the external environment.
Agentic AI can integrate generative AI
A crucial point to understand is that agentic AI and generative AI are not mutually exclusive. In fact, many agentic AI systems incorporate generative capabilities as one of their tools.
For example, an AI agent in a customer service context could use generative AI to write personalized responses while leveraging its agentic capabilities to decide when to escalate to a human. It could also employ knowledge base access tools to retrieve relevant information and advanced analysis functions to continuously evaluate customer satisfaction.
This synergy allows combining the best of both approaches: the creativity of generative AI and the decisional autonomy of agentic AI.
Agentic AI vs. AI agents: untangling the confusion
A frequent source of confusion in this field concerns the distinction between "agentic AI" and "AI agents." These terms are often used interchangeably, but they actually designate different concepts, although strongly related.
What is an AI agent?
An AI agent is an autonomous computer program designed to perform specific tasks by perceiving its environment, making decisions, and acting to achieve predefined objectives. It's essentially an individual software entity with a limited set of capabilities and objectives.
The AI agent is distinguished by its specialization, being designed to excel in a particular domain or task. Its limited autonomy gives it decision-making capability within a restricted framework. Integration also characterizes these agents, which can function alone or as components of a larger system. Finally, they possess a defined interface with clearly specified entry and exit points.
How AI agents integrate into agentic AI
Agentic AI can be considered as a paradigm or global approach, while AI agents are specific instances or components that embody this approach. To use an analogy:
Agentic AI is to the AI agent what architecture is to a building. A complete agentic AI system can comprise multiple AI agents working in synergy, each bringing its specific skills to the whole. In this configuration, each agent has distinct roles, capabilities, and objectives that contribute to the overall efficiency of the system.
In a practical context, a complex agentic AI system might orchestrate several specialized agents. A research agent might be responsible for collecting relevant information, while an analysis agent would process and interpret this data. A decision agent would then determine the most appropriate actions to undertake, and an execution agent would finally implement these actions in a concrete manner.
Common types of AI agents
In the current ecosystem, several types of AI agents can be distinguished based on their complexity and autonomy.
Reactive agents represent the most elementary level, responding directly to stimuli without long-term memory, like simple chatbots do. At a higher level, deliberative agents use reasoning and planning to make more nuanced decisions, like advanced recommendation systems.
In the communication sphere, interactive agents are specifically designed to communicate and collaborate with humans or other agents, like sophisticated virtual assistants do. Continuous evolution is at the heart of adaptive agents, which learn and improve over time through feedback, an essential quality of algorithmic trading agents.
Finally, at the most complex scale, multi-agent systems form networks of agents working together to achieve common or individual objectives, particularly useful in supply chain management systems.
Concrete applications: agentic AI vs. generative AI in action
To better understand the differences between agentic AI and generative AI, let's examine how they apply in several concrete domains.
AI in customer service
Generative AI approach: Generative AI in customer service excels at generating personalized responses to customer questions. It is particularly effective at creating content for FAQs and knowledge bases. Its flexibility also allows it to propose alternative formulations for customer communications, thus optimizing the user experience.
Agentic AI approach: Agentic AI takes customer service to the next level by analyzing customer intent and determining the best workflow for problem resolution. It can proactively access relevant systems such as CRM or order history without human intervention. Its intelligence allows it to decide when to escalate to a human agent for complex cases.
Beyond analysis, it can perform concrete actions such as order cancellation or reservation modification. One of its major assets lies in its ability to follow problems through to their complete resolution, thus offering a coherent and satisfying customer experience.
AI in Software development
Generative AI approach: In the field of software development, generative AI stands out for its ability to generate code from natural language descriptions, considerably simplifying developers' work. It also excels at proposing corrections for bugs identified in existing code. Its contribution extends to the creation of detailed technical documentation, facilitating the understanding and maintenance of systems.
Agentic AI approach: Agentic AI transforms software development by analyzing the context of a project to deeply understand its specific requirements. On this basis, it can propose software architectures adapted to the identified needs. Its analytical capability allows it to proactively detect potential problems in the code before they even become problematic.
Automation is at the heart of its functionalities, with the ability to automate tests and deployments to accelerate development cycles. Its global vision also allows it to monitor application performance and suggest relevant optimizations to improve system efficiency.
AI in Finance and investment
Generative AI approach: In the financial sector, generative AI stands out for its ability to generate detailed financial analysis reports that are accessible to various audiences. It also excels in creating personalized summaries of portfolio performance adapted to investors' profiles. Its aptitude for producing explanatory texts on market trends helps demystify complex financial concepts for a varied audience.
Agentic AI approach: Agentic AI revolutionizes finance by monitoring financial markets in real-time and instantly detecting anomalies and opportunities. It can identify investment opportunities based on predefined criteria with remarkable precision. Its autonomy allows it to automatically execute transactions according to established strategies without delay or emotion.
Its sophistication allows it to adjust asset allocations based on changing market conditions to optimize performance. Particularly valuable in periods of volatility, it can manage risk in real-time thanks to advanced protection mechanisms safeguarding investments.
AI in Healthcare
Generative AI approach: In the healthcare field, generative AI brings considerable value by generating summaries of medical records accessible to healthcare professionals. It excels in creating personalized educational content for patients, adapted to their condition and level of understanding. Its efficiency is also illustrated in the writing of preliminary medical reports, thus accelerating the clinical documentation process.
Agentic AI approach: Agentic AI transforms healthcare by continuously monitoring patients' vital signs to detect the slightest significant changes. Its reactivity allows it to automatically alert medical staff in case of detected anomalies, potentially vital. It brings increased precision by planning and adjusting medication doses according to established protocols and the patient's condition.
Its holistic vision allows it to coordinate care between different specialists, ensuring optimal treatment continuity. Its diagnostic impact is considerable, as it can aid in decision-making by simultaneously analyzing multiple sources of complex medical data.
These examples illustrate how agentic AI goes beyond simple content generation to embrace a more active and decisional role, often integrating generative capabilities as one of its many tools.
Multi-agent systems: the future of agentic AI
One of the most promising evolutions of agentic AI lies in multi-agent systems, where multiple specialized agents collaborate to solve complex problems.
Principles of multi-agent systems
A multi-agent system (MAS) is an AI approach where multiple autonomous agents interact in a shared environment. Each agent possesses its own capabilities, objectives, and perspectives, but they collaborate to achieve objectives that would be difficult or impossible to realize individually.
Specialization constitutes one of the pillars of MAS, with each agent focusing on specific tasks where it particularly excels. Collaboration represents the very essence of these systems, with agents constantly sharing information and coordinating their actions to maximize overall efficiency. A fascinating phenomenon of MAS is the emergence of systemic behaviors more sophisticated than those of the individual components.
Resilience represents a major advantage of this architecture, as the failure of one agent does not necessarily lead to the failure of the entire system, thus ensuring operational continuity. Finally, adaptability characterizes these systems, which can dynamically reconfigure their resources according to the changing needs of their environment.
Multi-agent system architectures
Several MAS architectures are currently being explored, each with its specific advantages. Hierarchical architecture relies on a "conductor" agent that methodically coordinates multiple specialized agents, ensuring global coherence of operations. In contrast, decentralized architecture privileges collaboration without central authority via shared protocols, offering more flexibility and resilience.
To combine the advantages of both previous approaches, hybrid architecture judiciously integrates hierarchical and decentralized elements according to the specific needs of the system, thus adapting to environments with varied requirements.
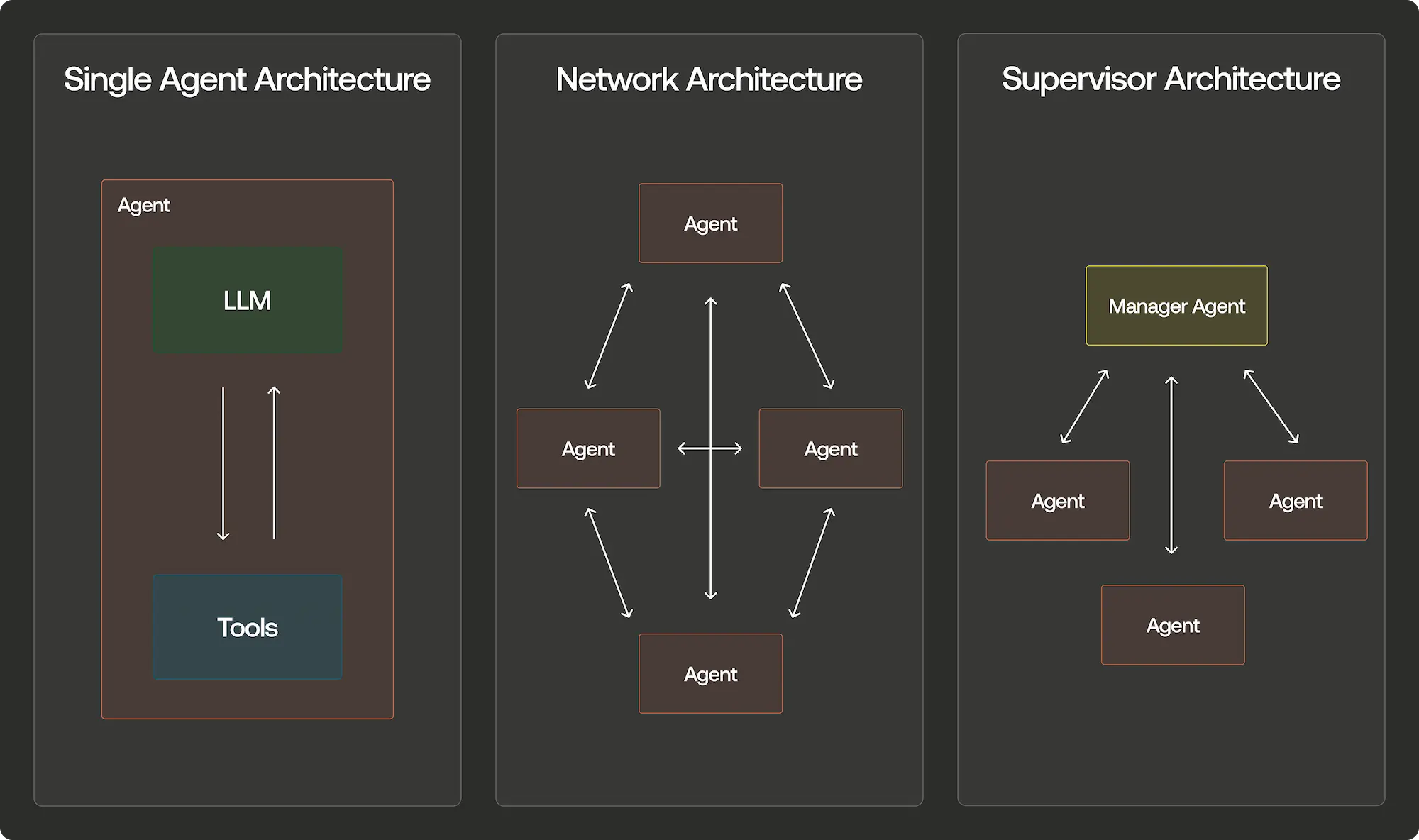
Examples of multi-agent systems in action
Multi-agent systems are beginning to appear in various sectors, revolutionizing traditional approaches. In business automation, specialized agents now manage different aspects of business operations, from data analysis to strategic decision-making, optimizing overall efficiency.
The supply chain also benefits from this approach, with agents who harmoniously coordinate supplies, production, logistics, and distribution to minimize delays and maximize profitability. In the field of scientific research, these systems accelerate discoveries by analyzing complex data, generating relevant hypotheses, and designing rigorous experiments.
Virtual environments are enriched thanks to autonomous agents that populate digital worlds, each with its own behavior and objectives, thus creating more realistic and immersive simulations.
A concrete example is Microsoft's AutoGen project, which allows creating teams of AI agents collaborating on complex tasks. A typical multi-agent system might include a research agent gathering relevant information, an analysis agent interpreting this data, a planning agent elaborating optimal strategies, a critic agent rigorously evaluating these strategies, and finally an execution agent precisely implementing the decided actions.
Challenges and limitations
Despite their revolutionary potential, agentic AI and multi-agent systems face several important challenges that must be addressed to allow their full adoption.
Technical challenges
Orchestration complexity constitutes one of the major challenges, as coordinating multiple agents and managing their interactions remains technically difficult, requiring sophisticated architectures. Goal alignment also represents a significant obstacle, as it is crucial to ensure that all agents in a system work toward coherent and complementary objectives.
Resource management poses complex optimization questions, particularly for the efficient allocation of computational resources among agents with different priorities. Finally, the security and robustness of these systems raise important concerns, requiring advanced protocols to protect them against vulnerabilities and unexpected behaviors.
Ethical and governance challenges
Transparency and explainability constitute major issues, as decisions made by multi-agent systems can prove difficult to explain, creating a problematic "black box." The question of responsibility also arises acutely: determining who is legally and morally responsible for actions undertaken by autonomous agents remains a legal and ethical challenge.
Risks related to bias and fairness are particularly concerning, as it is essential to avoid having biases present in training data influence the decisions made by these systems. Finally, human control remains a fundamental question, requiring the maintenance of an appropriate level of human supervision over these increasingly autonomous systems.
Current limitations
Domain knowledge currently represents a significant limitation, as current agents may lack specialized knowledge in certain technical or niche domains. Complex reasoning also remains a challenge, with the causal and counterfactual reasoning capabilities of these systems still limited when faced with highly abstract problems.
Social understanding constitutes a major obstacle, with current agents struggling to fully interpret social norms and cultural nuances essential in human interactions. Finally, efficient learning represents a crucial area for improvement, with the rapid acquisition of new skills from few examples remaining particularly difficult for these systems.
The future of agentic AI and multi-agent systems
Agentic AI represents a significant evolution compared to generative AI, and its development is rapidly accelerating. Several emerging trends will shape its future and its impact on our societies.
Convergence of approaches
We will likely witness a growing convergence between generative AI and agentic AI. Future systems will intelligently combine the strengths of both approaches to create more complete and versatile solutions.
This harmonious integration will combine the creativity and flexibility inherent to generative AI with the autonomy and action capability characteristic of agentic AI. The whole will be facilitated by the adoption of standards like the Model Context Protocol (MCP), which will considerably simplify integration with existing systems.
Personalization and specialization
AI agents will become increasingly personalized and specialized, adapting to specific contexts and needs. Personal agents will be meticulously adapted to the preferences and individual needs of each user, offering tailored assistance.
In the professional world, enterprise agents will be optimized for specific sectors and processes, considerably increasing productivity in targeted domains. For the most pointed needs, expert agents will master highly specialized knowledge domains, bringing professional-level expertise in precise niches.
Regulation and standardization
The evolution of agentic AI will also be shaped by regulatory and standardization considerations essential to its responsible development. The development of ethical frameworks specific to agentic AI will guide good practices and establish appropriate limits.
The emergence of industry standards for agent interoperability will facilitate their widespread adoption and allow better collaboration between different AI systems. Security certifications and evaluations for autonomous systems will become indispensable to establish the trust necessary for their large-scale deployment.
Finally, robust governance mechanisms for massive deployments of agentic AI will be put in place to ensure adequate supervision and responsible use of these powerful technologies.
Conclusion
Generative AI and agentic AI represent two complementary paradigms in the evolution of artificial intelligence. While generative AI has captured public attention with its ability to create impressive content, agentic AI could more profoundly transform our relationship with technology by introducing systems capable of acting in an autonomous and adaptive manner.
The distinction between agentic AI as a global paradigm and AI agents as specific components is essential to understand how these technologies will integrate into our organizations and society. Multi-agent systems, in particular, open the way to applications of unprecedented complexity and sophistication.
As these technologies continue to evolve, we will likely see a convergence between generative and agentic capabilities, creating systems that can both create and act with increasing autonomy. This evolution raises important questions about governance, ethics, and human control that will need to be addressed alongside technical advances.
For organizations and professionals, understanding these distinctions and following the rapid evolution of this field will be crucial to take advantage of new opportunities while effectively managing the associated risks.
FAQ on agentic AI and generative AI
What is the main difference between agentic AI and generative AI?
Generative AI creates content (text, images, code) in response to prompts, while agentic AI makes autonomous decisions and acts to achieve defined objectives, often without constant human intervention.
Is a model like GPT-4 or Claude an agentic AI?
In their standard form, GPT-4 and Claude are primarily generative models. However, when they are integrated into frameworks that allow them to use tools, access external data, and make sequential decisions, they can become components of an agentic AI system.
What is the relationship between AI agents and agentic AI?
Agentic AI is a paradigm or approach, while AI agents are specific components that embody this approach. An agentic AI system can comprise multiple AI agents working together to achieve common objectives.
Are multi-agent systems already used in industry?
Yes, multi-agent systems are beginning to be deployed in domains such as algorithmic finance, supply chain management, industrial automation, and some advanced virtual assistants. However, we are still in the early stages of their widespread adoption.
What are the main challenges of agentic AI compared to generative AI?
Agentic AI presents additional challenges in terms of security, governance, explainability, and control. As these systems can make autonomous decisions, ensuring they act in accordance with human intentions and ethical considerations becomes a major concern.
How can I start exploring agentic AI in my organization?
Start by identifying specific processes that could benefit from intelligent automation. Explore frameworks like AutoGen, LangChain, or solutions based on the Model Context Protocol (MCP). First develop simple single-objective agents before progressing to more complex systems.
Resources for going deeper
Want to learn more about agentic AI and AI agents? Check out our other articles on AI and discover how these technologies are transforming the digital landscape:
- Model Context Protocol (MCP): How this technology is revolutionizing artificial intelligence?
- Where is Webflow going with AI?
- ChatGPT 4o vs Midjourney: which is the best tool for generating AI images?
Want to stay informed about the latest advances in AI? Subscribe to our newsletter to receive exclusive analyses and expert advice directly in your inbox.